Artificial Intelligence (AI) is the simulation of human intelligence in machines programmed to think and learn. AI systems perform tasks that typically require human intelligence.
AI is transforming various industries by enhancing efficiency, accuracy, and productivity. Its applications range from healthcare to finance, providing innovative solutions to complex problems. Machine learning, a subset of AI, allows systems to learn from data and improve over time.
Businesses leverage AI for data analysis, predictive modeling, and automating repetitive tasks, thereby saving time and resources. Ethical considerations and data privacy remain crucial in AI development. As AI technology evolves, it promises to revolutionize how we live and work, offering unprecedented opportunities and challenges.
Introduction To AI Questionnaires
Artificial Intelligence (AI) questionnaires are tools to collect data about AI systems. These questionnaires help us understand the capabilities and limitations of AI. They also assess the ethical implications of AI use. Using AI questionnaires, developers can improve AI systems and ensure they are safe and fair.
Purpose And Importance
The main purpose of AI questionnaires is to gather detailed information. This information helps in evaluating AI systems. Here are some key reasons why AI questionnaires are important:
- Assessment: They assess the performance of AI systems.
- Improvement: They identify areas for improvement.
- Ethics: They ensure the ethical use of AI.
- Compliance: They help meet regulatory standards.
AI questionnaires are crucial for understanding AI’s impact. They help create better, safer AI systems. Organizations can use this data to make informed decisions about AI deployment.
Historical Background
The concept of AI questionnaires dates back to the early days of AI research. In the 1950s, Alan Turing proposed the Turing Test. This test aimed to evaluate a machine’s ability to exhibit intelligent behavior.
Over the years, AI questionnaires have evolved. Early questionnaires focused on basic AI capabilities. Today, they cover a wide range of aspects, including:
- Machine learning algorithms
- Data privacy and security
- Bias and fairness
- User interaction and experience
Modern AI questionnaires provide a comprehensive evaluation framework. They help ensure AI systems are robust, fair, and ethical.
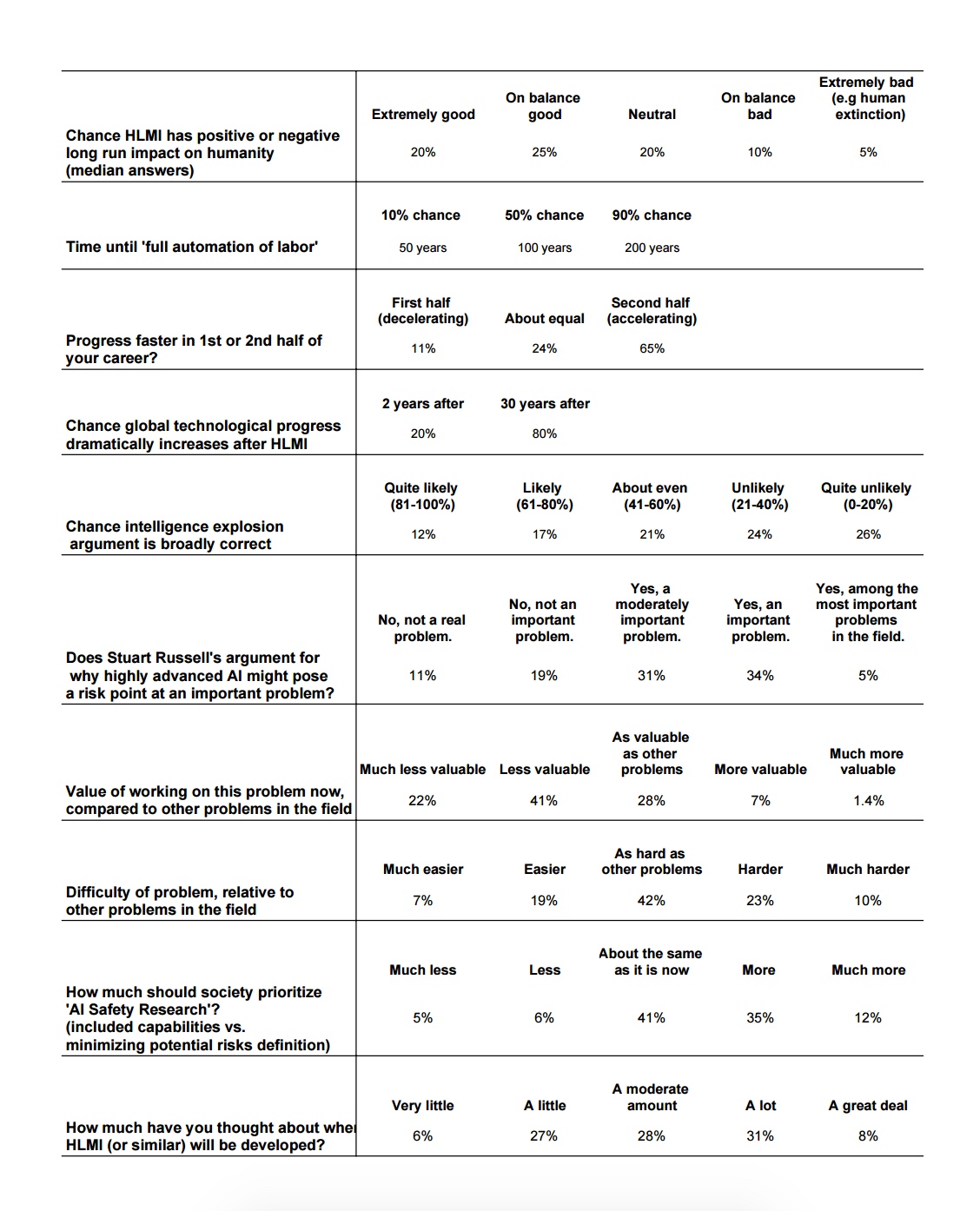
Credit: aiimpacts.org
Designing Effective AI Questionnaires
Creating an AI questionnaire is a vital task. It helps gather accurate data. This data is crucial for training AI models. A well-designed questionnaire ensures quality responses and reliable results.
Key Elements
Effective AI questionnaires have key elements. These elements guide the design process.
- Clarity: Use clear and simple language. Avoid jargon and complex terms.
- Relevance: Ask questions that are directly related to the AI objective.
- Length: Keep it short. Long questionnaires may lead to respondent fatigue.
- Types of Questions: Use a mix of open-ended and closed-ended questions.
Common Pitfalls
Designers often make mistakes in AI questionnaires. Avoid these common pitfalls for better outcomes.
- Ambiguity: Avoid vague questions. Make each question specific.
- Bias: Ensure questions are neutral. Avoid leading questions.
- Technical Issues: Test your questionnaire. Ensure it works on all devices.
- Overloading: Too many questions can overwhelm respondents. Keep it concise.
Types Of AI Questionnaires
Artificial Intelligence (AI) questionnaires are powerful tools. They help gather insights and data. There are different types of AI questionnaires. Each type serves a unique purpose.
Qualitative Vs Quantitative
Qualitative AI questionnaires focus on open-ended questions. They aim to collect detailed opinions and thoughts. These questionnaires help understand user experiences.
Quantitative AI questionnaires use closed-ended questions. They gather numerical data and statistics. These questionnaires provide measurable and comparable results.
Type | Purpose | Question Example |
---|---|---|
Qualitative | Gather detailed opinions | What do you think about AI in healthcare? |
Quantitative | Collect numerical data | Rate your satisfaction with AI tools (1-10). |
Industry-specific Applications
AI questionnaires are tailored for different industries. Each industry has unique needs and goals.
- Healthcare: Assess patient satisfaction, and gather feedback on AI diagnostics.
- Finance: Evaluate customer trust in AI-driven financial advice.
- Education: Measure student engagement with AI learning tools.
These industry-specific applications help fine-tune AI systems. They ensure AI tools meet industry standards and expectations.
Data Collection Methods
Gathering data is crucial for any artificial intelligence questionnaire. Different methods offer various advantages. Below, we’ll explore some popular data collection methods.
Online Surveys
Online surveys are a popular method for collecting data. They reach a large audience quickly. They are also cost-effective.
- Simple to distribute
- Easy to analyze
- Respondents can complete them at their convenience
Online surveys can include multiple types of questions:
Question Type | Example |
---|---|
Multiple Choice | What is your favorite color? (Red, Blue, Green) |
Open-Ended | Describe your experience with our product. |
Rating Scale | Rate our service from 1 to 5. |
Interviews And Focus Groups
Interviews provide in-depth information. They allow for follow-up questions. This method is time-consuming but offers rich data.
Focus groups gather people for a discussion. They capture different viewpoints. This method also provides qualitative insights.
- In-depth understanding of subjects
- Opportunity to ask follow-up questions
- Can reveal group dynamics
Both methods help understand complex issues. They are useful for exploratory research.
Analyzing Ai Questionnaire Data
Analyzing AI questionnaire data is crucial. It helps us understand the effectiveness of AI systems. This involves using various statistical and machine-learning techniques. The objective is to derive meaningful insights from the collected data.
Statistical Tools
Statistical tools play a vital role in data analysis. They help in summarizing and interpreting the data effectively. Here are some common tools used:
- Descriptive Statistics: Mean, median, and mode help in understanding data distribution.
- Inferential Statistics: These include t-tests and chi-square tests. They help in making predictions or inferences about a population based on sample data.
- Regression Analysis: Helps in identifying relationships between variables.
Using these tools, we can identify patterns and trends. This is essential for making data-driven decisions.
Machine Learning Techniques
Machine learning techniques are also crucial. They help in predicting outcomes and identifying hidden patterns. Here are some commonly used techniques:
- Supervised Learning: This includes algorithms like linear regression and decision trees. They are used for predicting outcomes based on labeled data.
- Unsupervised Learning: Techniques like clustering and principal component analysis (PCA). They help in identifying hidden patterns in unlabeled data.
- Reinforcement Learning: This involves training models based on feedback from their actions. It is widely used in robotics and game theory.
Machine learning techniques enhance the accuracy of data analysis. They provide deeper insights and predictive capabilities.
Combining Statistical Tools And Machine Learning Techniques
Combining statistical tools with machine learning techniques offers a comprehensive approach to data analysis. This combination leverages the strengths of both methods. It ensures robust and accurate results. Here’s a comparison:
Statistical Tools | Machine Learning Techniques |
---|---|
Summarizes data | Predicts outcomes |
Identifies relationships | Finds hidden patterns |
Uses sample data | Handles large datasets |
This approach ensures a holistic understanding of AI questionnaire data. It aids in making informed decisions based on comprehensive analysis.
Trends In AI Questionnaire Usage
Artificial Intelligence (AI) Questionnaires are transforming data collection. They offer precise insights and streamline decision-making. Let’s explore the trends shaping AI questionnaire usage.
Emerging Technologies
AI is leveraging emerging technologies to enhance questionnaire efficiency. These technologies include:
- Natural Language Processing (NLP): Improves understanding of user responses.
- Machine Learning (ML): Enables adaptive questioning.
- Chatbots: Facilitates real-time interaction.
NLP helps AI understand human language better. ML allows questionnaires to adapt based on responses. Chatbots provide instant feedback and engagement.
Adoption Across Sectors
AI questionnaires are being adopted across various sectors. Key sectors include:
Sector | Application |
---|---|
Healthcare | Patient surveys and diagnostics |
Education | Student assessments |
Retail | Customer feedback |
Finance | Client risk assessments |
In healthcare, AI questionnaires collect patient data efficiently. In education, they assess student performance accurately. Retail uses them for valuable customer feedback. Finance sectors use them for precise client assessments.
Case Studies
Exploring real-world applications of Artificial Intelligence (AI) can provide valuable insights. Case studies reveal the practical benefits and challenges of AI. Let’s dive into some compelling examples.
Successful Implementations
Several industries have successfully implemented AI solutions. These cases highlight the transformative impact of AI.
- Healthcare: AI-powered diagnostic tools help doctors identify diseases early. These tools analyze medical images and patient data.
- Finance: Fraud detection systems use AI to monitor transactions. They quickly flag suspicious activities, protecting users and banks.
- Retail: Personalized recommendation engines enhance customer experience. AI suggests products based on past purchases and browsing history.
Lessons Learned
From these successful implementations, important lessons emerge. These lessons guide future AI projects.
- Data Quality: High-quality data is crucial for effective AI. Ensure data is clean, accurate, and relevant.
- Human-AI Collaboration: AI should complement human skills. Combining AI insights with human judgment leads to better outcomes.
- Continuous Improvement: AI systems need regular updates. Continuous learning and improvement enhance their performance.
Industry | AI Application | Benefit |
---|---|---|
Healthcare | Diagnostic Tools | Early disease detection |
Finance | Fraud Detection | Protects users and banks |
Retail | Recommendation Engines | Enhanced customer experience |

Credit: www.researchgate.net
Future Of AI Questionnaires
The future of AI questionnaires holds great promise. With rapid advancements, these tools are becoming smarter and more efficient. They can now understand user intent better and provide more accurate responses. This section explores the future of AI questionnaires.
Predicted Developments
Experts predict several exciting developments in AI questionnaires.
- Personalization: AI will tailor questions to individual users.
- Natural Language Processing: Improved NLP will enhance understanding.
- Real-Time Analysis: Instant feedback will become more common.
- Integration: Seamless integration with other systems is expected.
Potential Challenges
Despite the benefits, AI questionnaires face some challenges.
- Data Privacy: Ensuring user data remains secure is crucial.
- Bias: Reducing bias in AI algorithms is essential.
- Complexity: Managing complex queries can be difficult.
- Cost: Developing advanced AI solutions can be expensive.
Understanding these challenges helps improve AI questionnaires. It ensures they remain effective and beneficial for users.

Credit: www.sciencedirect.com
Frequently Asked Questions
What Is Artificial Intelligence?
Artificial intelligence (AI) refers to machines mimicking human intelligence. It involves learning, reasoning, and problem-solving. AI can process large amounts of data quickly. It enhances decision-making and automates tasks.
How Does AI Work?
AI uses algorithms and machine learning. It analyzes data patterns to make decisions. Training data helps AI improve over time. AI systems learn from new data continuously.
What Are The Types Of AI?
There are three main types: Narrow AI, General AI, and Superintelligent AI. Narrow AI specializes in specific tasks. General AI can perform any human task. Superintelligent AI surpasses human intelligence.
What Are The Applications Of AI?
AI is used in various fields. It includes healthcare, finance, and customer service. AI improves diagnostics, automates trading, and enhances user experiences. AI also powers virtual assistants like Siri and Alexa.
Conclusion
AI questionnaires offer valuable insights into user preferences and behaviors. They help businesses make data-driven decisions. Embrace AI technology to enhance customer experience and improve operational efficiency. Stay ahead by integrating AI questionnaires into your strategy. This will ensure your business remains competitive and innovative in the rapidly evolving digital landscape.